SPREAD: A Large-scale, High-fidelity Synthetic Dataset for Multiple Forest Vision Tasks
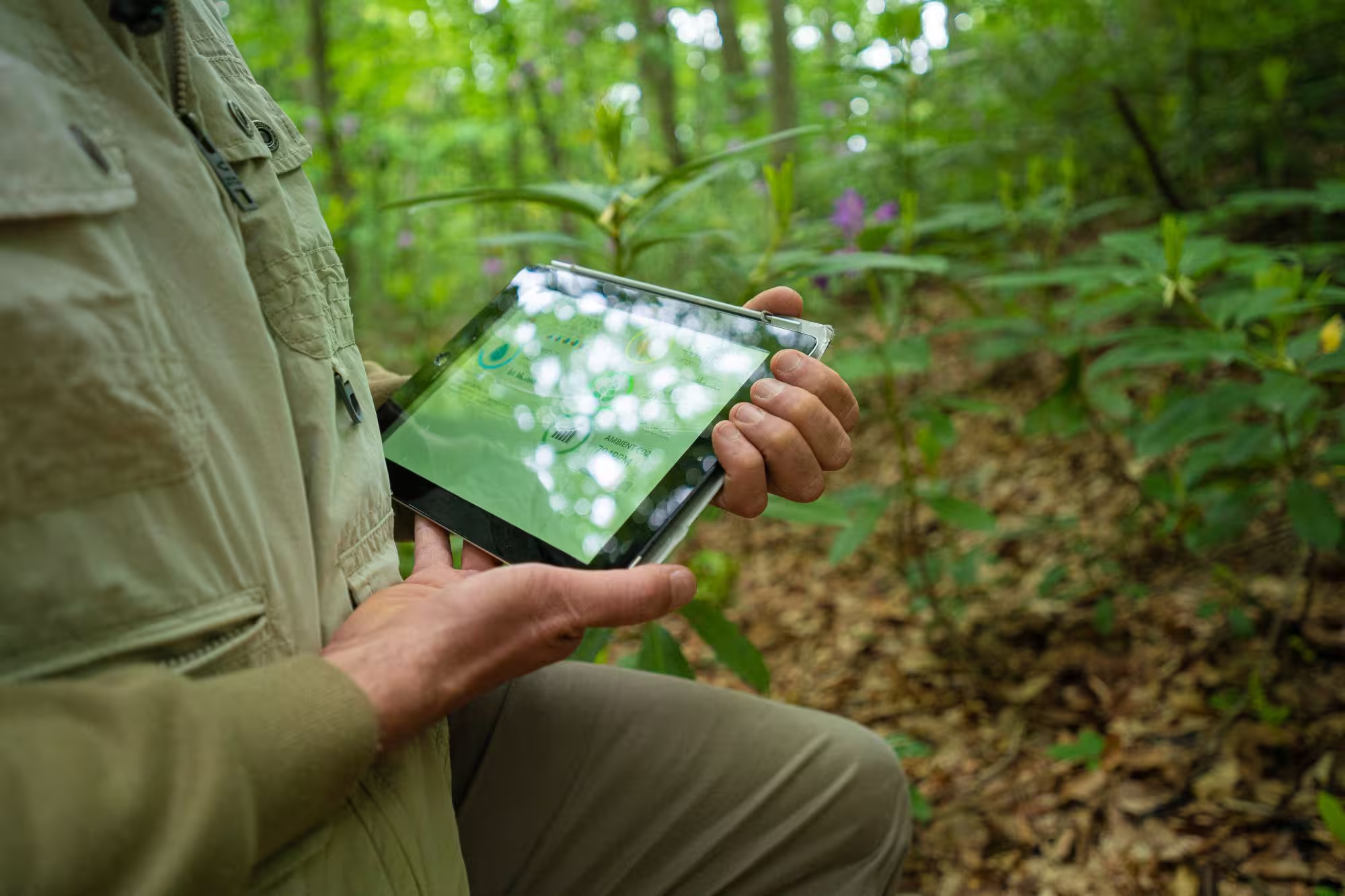
To develop research that provides impactful solutions to the urgent challenges of climate change, access to comprehensive and high-quality data is essential. Machine learning is pivotal in this effort, offering the capability to analyze vast and complex datasets, uncover hidden patterns, and generate accurate predictions that guide effective mitigation and adaptation strategies. The project has established the Synthetic Photo-realistic Arboreal Dataset (SPREAD), a state-of-the-art synthetic dataset specifically designed for forest-related machine-learning tasks.
Developed using Unreal Engine 5, SPREAD goes beyond existing synthetic forest datasets in terms of realism, diversity, and comprehensiveness. It includes RGB, depth images, point clouds, semantic and instance segmentation labels, along with key parameters such as tree ID, location, diameter at breast height (DBH), height, and canopy diameter. In exemplary experiments, it was found that SPREAD significantly reduces the need to use real-world datasets for trunk segmentation tasks and enhances model segmentation performance.